Magento 2 and AI: Practical Use Cases for E-Commerce
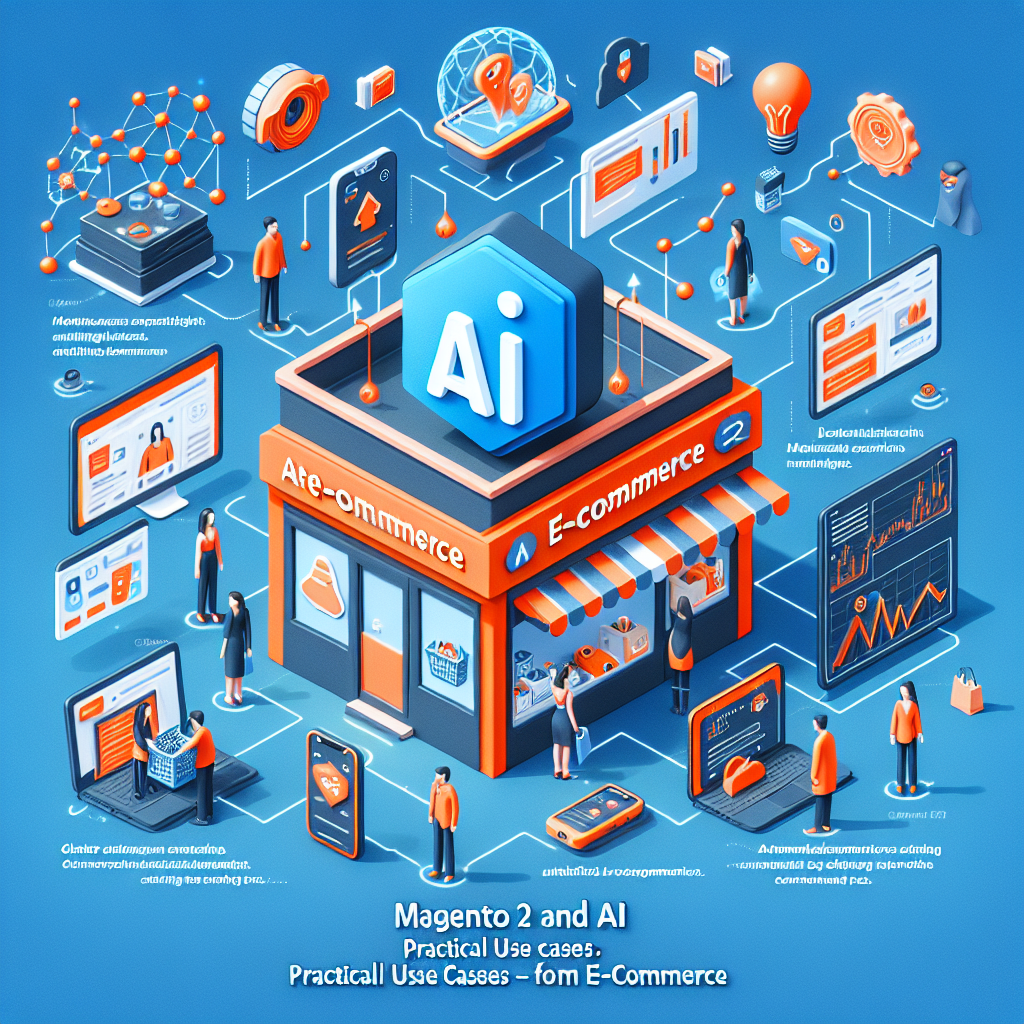
Magento 2 and AI: Practical Use Cases for E-Commerce
Artificial Intelligence (AI) is no longer a futuristic concept—it's here, and it's transforming e-commerce in ways we couldn't have imagined a few years ago. If you're running a Magento 2 store, integrating AI can give you a competitive edge by automating processes, personalizing experiences, and optimizing operations.
In this post, we'll explore practical AI use cases for Magento 2, complete with code snippets and implementation steps to help you get started. Whether you're a developer or a store owner, these insights will help you harness AI's power effectively.
Why AI in Magento 2?
AI can analyze vast amounts of data, predict trends, and automate repetitive tasks—making it perfect for e-commerce. From product recommendations to fraud detection, AI-powered tools can enhance customer experience and streamline backend operations.
1. Personalized Product Recommendations
One of the most effective AI applications in e-commerce is personalized recommendations. Instead of showing generic "best sellers," AI analyzes browsing behavior, purchase history, and customer preferences to suggest highly relevant products.
Implementation Steps:
Option 1: Using a Pre-built Extension
Extensions like MagePlaza AI Recommendations or Klevu Smart Search integrate seamlessly with Magento 2 and require minimal setup.
Option 2: Custom Implementation with TensorFlow
If you prefer a custom solution, you can use TensorFlow.js to build a recommendation engine. Here’s a basic example:
// Example: Training a simple recommendation model
const tf = require('@tensorflow/tfjs-node');
// Sample customer-product interaction data
const interactions = [
{ customerId: 1, productId: 101, rating: 5 },
{ customerId: 1, productId: 102, rating: 3 },
{ customerId: 2, productId: 101, rating: 4 },
{ customerId: 2, productId: 103, rating: 5 },
];
// Convert data to tensors
const customerIds = interactions.map(i => i.customerId);
const productIds = interactions.map(i => i.productId);
const ratings = interactions.map(i => i.rating);
const customerTensor = tf.tensor1d(customerIds, 'int32');
const productTensor = tf.tensor1d(productIds, 'int32');
const ratingTensor = tf.tensor1d(ratings, 'float32');
// Define and train a simple collaborative filtering model
const model = tf.sequential();
model.add(tf.layers.dense({ units: 10, inputShape: [2] }));
model.add(tf.layers.dense({ units: 1 }));
model.compile({ optimizer: 'adam', loss: 'meanSquaredError' });
await model.fit(
tf.stack([customerTensor, productTensor], 1),
ratingTensor,
{ epochs: 100 }
);
// Use the model to predict ratings for a customer
const predictions = model.predict(tf.stack([
tf.tensor1d([1, 1, 1], 'int32'), // Customer 1
tf.tensor1d([101, 102, 103], 'int32') // Products
], 1));
predictions.print();
Once trained, you can use this model in a Magento 2 block to display personalized recommendations.
2. AI-Powered Search (Smart Search)
Traditional search functions often fail when customers use vague or misspelled queries. AI-enhanced search understands intent, corrects typos, and delivers more accurate results.
Implementation Steps:
Option 1: Using Elasticsearch with AI Plugins
Magento 2 supports Elasticsearch, which can be enhanced with NLP (Natural Language Processing) plugins like Elasticsearch Learning to Rank.
# Example: Configuring Elasticsearch with Magento 2
bin/magento config:set catalog/search/engine elasticsearch7
bin/magento config:set catalog/search/elasticsearch7_server_hostname localhost
bin/magento config:set catalog/search/elasticsearch7_server_port 9200
bin/magento indexer:reindex catalogsearch_fulltext
Option 2: Custom Implementation with BERT
For advanced semantic search, you can integrate Google's BERT model:
// Example: Using TensorFlow.js with BERT for semantic search
const model = await tf.loadGraphModel('https://tfhub.dev/tensorflow/tfjs-model/bert_en_uncased_L-12_H-768_A-12/1/default/1');
const query = "comfortable running shoes";
const products = ["Nike Air Zoom Pegasus", "Adidas Ultraboost", "Puma Velocity Nitro"];
// Encode query and products
const inputs = {
"input_ids": tf.tensor2d([[101, ...query.split(' ').map(token => tokenizer(token)), 102]]),
"attention_mask": tf.tensor2d([[1, 1, 1, 1, 1, 1]]),
"token_type_ids": tf.tensor2d([[0, 0, 0, 0, 0, 0]])
};
const queryEmbedding = model.predict(inputs).pooled_output;
// Compare with product embeddings to find best matches
const similarities = products.map(product => {
const productEmbedding = /* generate embedding for product */;
return tf.dot(queryEmbedding, productEmbedding).dataSync()[0];
});
console.log("Best match:", products[similarities.indexOf(Math.max(...similarities))]);
3. Dynamic Pricing Optimization
AI can analyze market trends, competitor pricing, and demand fluctuations to suggest optimal pricing strategies in real-time.
Implementation Steps:
// Example: Simple price optimization algorithm
function optimizePrice(productId, currentPrice) {
const demandHistory = getDemandData(productId);
const competitorPrices = getCompetitorPrices(productId);
const elasticity = calculatePriceElasticity(demandHistory);
// Simple rule-based adjustment (replace with ML model in production)
const avgCompetitorPrice = competitorPrices.reduce((a,b) => a+b, 0) / competitorPrices.length;
const demandTrend = getTrend(demandHistory);
let newPrice = currentPrice;
if (demandTrend > 0.1 && currentPrice < avgCompetitorPrice) {
newPrice = currentPrice * 1.05; // Increase price if demand is growing and we're below competitors
} else if (demandTrend < -0.1 || currentPrice > avgCompetitorPrice * 1.1) {
newPrice = currentPrice * 0.95; // Decrease price if demand is falling or we're significantly above competitors
}
return Math.max(newPrice, getMinimumPrice(productId)); // Ensure we don't go below cost
}
// In Magento 2 observer:
$event->getProduct()->setPrice(optimizePrice(
$event->getProduct()->getId(),
$event->getProduct()->getPrice()
));
4. AI-Powered Fraud Detection
E-commerce stores lose billions to fraud annually. AI can analyze patterns in orders to detect potentially fraudulent transactions before they're processed.
Implementation Steps:
// Example: Fraud detection using a pre-trained model
const fraudModel = await tf.loadLayersModel('path/to/fraud-detection-model.json');
function analyzeOrderForFraud(order) {
const features = [
order.getShippingAddress().distanceFromBilling(),
order.getCustomer().getOrderHistory().length,
order.getTotal(),
order.getShippingMethod() === 'express' ? 1 : 0,
order.getBillingAddress().matchesShipping() ? 0 : 1
];
const prediction = fraudModel.predict(tf.tensor2d([features]));
return prediction.dataSync()[0] > 0.7; // Threshold for fraud probability
}
// In Magento 2 payment processor:
if (analyzeOrderForFraud($order)) {
$payment->setIsFraudDetected(true);
$payment->setIsTransactionPending(true);
}
5. Chatbots for Customer Support
AI-powered chatbots can handle common customer queries 24/7, reducing support costs and improving response times.
Implementation Steps:
Option 1: Dialogflow Integration
// Example: Dialogflow webhook for Magento 2
app.post('/dialogflow-webhook', (req, res) => {
const intent = req.body.queryResult.intent.displayName;
const parameters = req.body.queryResult.parameters;
if (intent === 'TrackOrder') {
const order = loadOrder(parameters.orderId);
res.json({
fulfillmentText: `Your order #${parameters.orderId} is ${order.getStatus()}`
});
}
// Handle other intents...
});
Option 2: Custom Transformer-based Chatbot
// Example: Simple chatbot with TensorFlow.js
const model = await tf.loadLayersModel('path/to/chatbot-model.json');
const tokenizer = /* load your tokenizer */;
function respondToCustomer(query) {
const input = tokenizer.encode(query);
const responseTokens = model.predict(tf.tensor2d([input]));
return tokenizer.decode(responseTokens.argMax(-1).dataSync());
}
6. Visual Search and Image Recognition
Allow customers to search your catalog by uploading images—perfect for fashion, furniture, and other visually distinctive products.
Implementation Steps:
// Example: Using TensorFlow.js for visual search
const mobilenet = await tf.loadLayersModel('https://storage.googleapis.com/tfjs-models/tfjs/mobilenet_v1_0.25_224/model.json');
async function findSimilarProducts(imageElement) {
// Preprocess the image
const imageTensor = tf.browser.fromPixels(imageElement)
.resizeNearestNeighbor([224, 224])
.toFloat()
.expandDims();
// Extract features
const features = mobilenet.predict(imageTensor);
// Compare with product images in database
const similarProducts = await database.query(
'SELECT product_id FROM product_embeddings ORDER BY embedding <=> $1 LIMIT 5',
[features.dataSync()]
);
return similarProducts;
}
// In Magento 2 frontend:
document.getElementById('upload').addEventListener('change', (e) => {
const img = document.createElement('img');
img.src = URL.createObjectURL(e.target.files[0]);
img.onload = () => {
findSimilarProducts(img).then(products => {
// Display similar products
});
};
});
7. Predictive Inventory Management
AI can forecast demand to optimize inventory levels, reducing both stockouts and overstock situations.
Implementation Steps:
// Example: Time series forecasting for inventory
const model = tf.sequential();
model.add(tf.layers.lstm({ units: 50, inputShape: [10, 1] }));
model.add(tf.layers.dense({ units: 1 }));
model.compile({ optimizer: 'adam', loss: 'meanSquaredError' });
// Train on historical sales data
const salesHistory = /* array of daily sales quantities */;
const xs = tf.tensor3d(
salesHistory.slice(0, -10).map((_, i) =>
salesHistory.slice(i, i + 10).map(x => [x])
);
const ys = tf.tensor2d(salesHistory.slice(10), [salesHistory.length - 10, 1]);
await model.fit(xs, ys, { epochs: 100 });
// Predict next week's demand
const lastWeek = tf.tensor3d([salesHistory.slice(-10).map(x => [x])]);
const nextWeekDemand = model.predict(lastWeek).dataSync()[0];
// In Magento 2 inventory management:
$stockItem->setQty(Math.max(nextWeekDemand * 1.2, $minimumStockLevel));
8. Sentiment Analysis for Product Reviews
Automatically analyze customer reviews to gauge satisfaction and identify common issues.
Implementation Steps:
// Example: Sentiment analysis with TensorFlow.js
const sentimentModel = await tf.loadLayersModel('https://storage.googleapis.com/tfjs-models/tfjs/sentiment_cnn_v1/model.json');
const metadata = await fetch('https://storage.googleapis.com/tfjs-models/tfjs/sentiment_cnn_v1/metadata.json').then(r => r.json());
function analyzeSentiment(text) {
const input = padSequences([text.split(' ').map(word => {
return metadata.word_index[word] + metadata.index_from;
}], metadata.max_len);
const prediction = sentimentModel.predict(tf.tensor2d(input, [1, metadata.max_len]));
return prediction.dataSync()[0]; // 0-1 where >0.6 is positive
}
// In Magento 2 review save observer:
$sentiment = analyzeSentiment($review->getDetail());
$review->setData('sentiment_score', $sentiment);
if ($sentiment < 0.3) {
$this->alertCustomerService($review);
}
Getting Started with AI in Magento 2
Ready to implement AI in your Magento 2 store? Here's a quick roadmap:
- Identify Pain Points: Start with areas where AI can have the most impact (search, recommendations, fraud detection).
- Choose Your Approach: Decide between pre-built solutions (extensions/SaaS) or custom implementations.
- Prepare Your Data: Clean and structure your data for AI training (product catalogs, customer behavior, transaction history).
- Start Small: Implement one AI feature at a time, measure results, then expand.
- Monitor and Optimize: Continuously track performance and refine your models.
AI is transforming e-commerce, and Magento 2 stores that embrace these technologies early will gain a significant competitive advantage. Whether you start with simple product recommendations or dive into advanced computer vision applications, the key is to begin experimenting today.
Have you implemented AI in your Magento store? Share your experiences in the comments!